Population Risk Tools
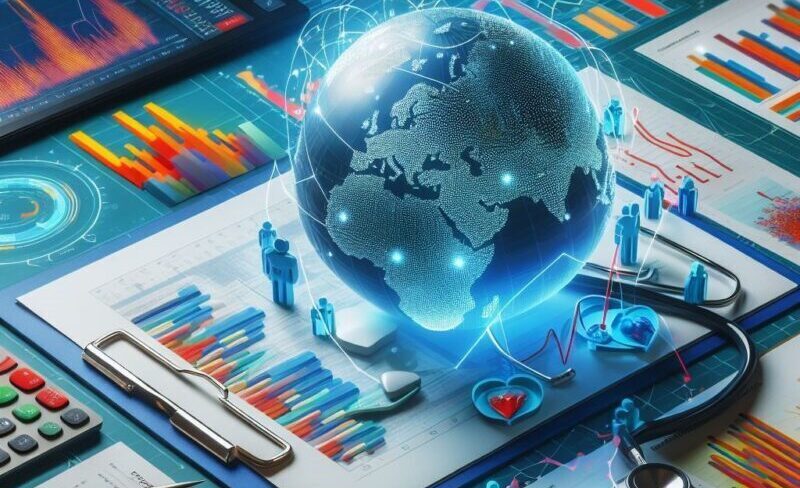
Powered by DALL·E 3
Diabetes Population Risk Tool (DPoRT)
10-year incidence of physician-diagnosed Type 2 diabetes.
Abstract
Background: National estimates of the upcoming diabetes epidemic are needed to understand the distribution of diabetes risk in the population and to inform health policy.
Objective: To create and validate a population-based risk prediction tool for incident diabetes using commonly collected national survey data.
Methods: With the use of a cohort design that links baseline risk factors to a validated population-based diabetes registry, a model (Diabetes Population Risk Tool (DPoRT)) was developed to predict 9-year risk for diabetes. The probability of developing diabetes was modelled using sex-specific Weibull survival functions for people >20 years of age without diabetes (N=19 861). The model was validated in two external cohorts in Ontario (N=26 465) and Manitoba (N=9899). Predictive accuracy and model performance were assessed by comparing observed diabetes rates with predicted estimates. Discrimination and calibration were measured using a C statistic and Hosmer–Lemeshow χ2 statistic (χ2H–L).
Results: Predictive factors included were body mass index, age, ethnicity, hypertension, immigrant status, smoking, education status and heart disease. DPoRT showed good discrimination (C=0.77–0.80) and calibration (χ2H–L <20) in both external validation cohorts.
Conclusions: This algorithm can be used to estimate diabetes incidence and quantify the effect of interventions using routinely collected survey data.
Link to research report: Rosella LC, Manuel DG, Burchill C, Stukel TA; PHIAT-DM team. A population-based risk algorithm for the development of diabetes: development and validation of the Diabetes Population Risk Tool (DPoRT). J Epidemiol Community Health. 2011 Jul;65(7):613-20. doi: 10.1136/jech.2009.102244. Epub 2010 Jun 1. PMID: 20515896; PMCID: PMC3112365. https://doi.org/10.1136/jech.2009.102244.
Chronic Disease Population Risk Tool (CDPoRT)
10-year first incidence of six major chronic diseases (myocardial infarction, chronic obstructive pulmonary disorder, congestive heart failure, diabetes, stroke, and lung cancer).
Abstract
Importance: Predicting chronic disease incidence for the population provides a comprehensive picture to health policy makers of their jurisdictions’ overall future chronic disease burden. However, no population-based risk algorithm exists for estimating the risk of first major chronic disease.
Objective: To develop and validate the Chronic Disease Population Risk Tool (CDPoRT), a population risk algorithm that predicts the 10-year incidence of the first major chronic disease in the adult population.
Design, Setting, and Participants: In this cohort study, CDPoRT was developed and validated with 6 cycles of the Canadian Community Health Survey, linked to administrative data from January 2000 to December 2014. Development and internal validation (bootstrap and split sample) of CDPoRT occurred in Ontario, Canada, from June 2018 to April 2019 followed by external validation in Manitoba from May 2019 to July 2019. The study cohorts included 133 991 adults (≥20 years) representative of the Ontario and Manitoba populations who did not have a history of major chronic disease.
Exposures: Predictors were routinely collected risk factors from the Canadian Community Health Survey, such as sociodemographic factors (eg, age), modifiable lifestyle risk factors (i.e., alcohol consumption, cigarette smoking, unhealthy diet, and physical inactivity), and other health-related factors (eg, body mass index).
Main Outcomes and Measures: Six major chronic diseases were considered, as follows: congestive heart failure, chronic obstructive pulmonary disease, diabetes, myocardial infarction, lung cancer, and stroke. Sex-specific CDPoRT algorithms were developed with a Weibull model. Model performance was evaluated with measures of overall predictive performance (eg, Brier score), discrimination (eg, Harrell C index), and calibration (eg, calibration curves).
Results: The Ontario cohort (n = 118 747) was younger (mean [SD] age, 45.6 [16.1] vs 46.3 [16.4] years), had more immigrants (23 808 [20.0%] vs 1417 [10.7%]), and had a lower mean (SD) body mass index (26.9 [5.1] vs 27.7 [5.4]) than the Manitoba cohort (n = 13 244). During development, the full and parsimonious CDPoRT models had similar Brier scores (women, 0.087; men, 0.091), Harrell C index values (women, 0.779; men, 0.783), and calibration curves. A simple version consisting of cigarette smoking, age, and body mass index performed slightly worse than the other versions (eg, Brier score for women, 0.088; for men, 0.092). Internal validation showed consistent performance across models, and CDPoRT performed well during external validation. For example, the female parsimonious version had C index values for bootstrap, split sample, and external validation of 0.778, 0.776, and 0.752, respectively.
Conclusions and Relevance: In this study, CDPoRT provided accurate, population-based risk estimates for the first major chronic disease.
Link to research article:Ng R, Sutradhar R, Kornas K, Wodchis WP, Sarkar J, Fransoo R, Rosella LC. Development and Validation of the Chronic Disease Population Risk Tool (CDPoRT) to Predict Incidence of Adult Chronic Disease. JAMA Netw Open. 2020 Jun 1;3(6):e204669. doi:10.1001/jamanetworkopen.2020.4669. PMID: 32496565; PMCID: PMC7273197.
High Resource User Population Risk Tool (HRUPoRT)
5-year incidence of becoming a high health care resource user (top 5%).
Abstract
Abstract Predicting high healthcare resource users is important for informing prevention strategies and healthcare decision-making. We aimed to cross-provincially validate the High Resource User Population Risk Tool (HRUPoRT), a predictive model that uses population survey data to estimate 5 year risk of becoming a high healthcare resource user. The model, originally derived and validated in Ontario, Canada, was applied to an external validation cohort. HRUPoRT model predictors included chronic conditions, socio-demographics, and health behavioural risk factors. The cohort consisted of 10,504 adults (≥18 years old) from the Canadian Community Health Survey in Manitoba, Canada (cycles 2007/08 and 2009/10). A person-centred costing algorithm was applied to linked health administrative databases to determine respondents’ healthcare utilization over 5 years. Model fit was assessed using the c-statistic for discrimination and calibration plots. In the external validation cohort, HRUPoRT demonstrated strong discrimination (c statistic = 0.83) and was well calibrated across the range of risk. HRUPoRT performed well in an external validation cohort, demonstrating transportability of the model in other jurisdictions. HRUPoRT’s use of population survey data enables a health equity focus to assist with decision-making on prevention of high healthcare resource use.
Link to research report: Rosella LC, Kornas K, Sarkar J, Fransoo R. External Validation of a Population-Based Prediction Model for High Healthcare Resource Use in Adults. Healthcare 2020, 8, 537. https://doi.org/10.3390/healthcare8040537
Obesity Population Risk Tool (OPoRT)
Abstract
Background: Given the dramatic rise in the prevalence of obesity, greater focus on prevention is necessary. We sought to develop and validate a population risk tool for obesity to inform prevention efforts.
Methods: We developed the Obesity Population Risk Tool (OPoRT) using the longitudinal National Population Health Survey and sex-specific Generalized Estimating Equations to predict the 10-year risk of obesity among adults 18 and older. The model was validated using a bootstrap approach accounting for the survey design. Model performance was measured by the Brier statistic, discrimination was measured by the C-statistic, and calibration was assessed using the Hosmer-Lemeshow Goodness of Fit Chi Square (HL χ2).
Results: Predictive factors included baseline body mass index, age, time and their interactions, smoking status, living arrangements, education, alcohol consumption, physical activity, and ethnicity. OPoRT showed good performance for males and females (Brier 0.118 and 0.095, respectively), excellent discrimination (C statistic ≥ 0.89) and achieved calibration (HL χ2 <20).
Conclusion: OPoRT is a valid and reliable algorithm that can be applied to routinely collected survey data to estimate the risk of obesity and identify groups at increased risk of obesity. These results can guide prevention efforts aimed at reducing the population burden of obesity.
Link to research article: Lebenbaum M, Espin-Garcia O, Li Y, Rosella LC. Development and validation of a population based risk algorithm for obesity: The Obesity Population Risk Tool (OPoRT). PLoS One. 2018 Jan 18;13(1):e0191169. doi: 10.1371/journal.pone.0191169. PMID: 29346391; PMCID: PMC5773177. https://doi.org/10.1371/journal.pone.0191169.
Premature Mortality Population Risk Tool (PreMPoRT)
5-year incidence of premature mortality (death prior to age 75).
Abstract
Introduction To develop and validate the Premature Mortality Population Risk Tool (PreMPoRT), a population-based risk algorithm that predicts the 5-year incidence of premature mortality among the Canadian adult population.
Methods Retrospective cohort analysis used six cycles of the Canadian Community Health Survey linked to the Canadian Vital Statistics Database (2000–2017). The cohort comprised 500 870 adults (18–74 years). Predictors included sociodemographic factors, self-perceived measures, health behaviours and chronic conditions. Three models (minimal, primary and full) were developed. PreMPoRT was internally validated using a split set approach and externally validated across three hold-out cycles. Performance was assessed based on predictive accuracy, discrimination and calibration.
Results The cohort included 267 460 females and 233 410 males. Premature deaths occurred in 1.40% of females and 2.05% of males. Primary models had 12 predictors (females) and 13 predictors (males). Shared predictors included age, income quintile, education, self-perceived health, smoking, emphysema/chronic obstructive pulmonary disease, heart disease, diabetes, cancer and stroke. Male-specific predictors were marital status, Alzheimer’s disease and arthritis while female-specific predictors were body mass index and physical activity. External validation cohort differed slightly in demographics. Female model performance: split set (c-statistic: 0.852), external (c-statistic: 0.856). Male model performance: split set and external (c-statistic: 0.846). Calibration showed slight overprediction for high-risk individuals and good calibration in key subgroups.
Conclusions PreMPoRT achieved the strongest discrimination and calibration among existing prediction models for premature mortality. The model produces reliable estimates of future incidence of premature mortality and may be used to identify subgroups who may benefit from public health interventions.
Link to research article: O’Neill M, Hurst M, Pagalan L, Diemert LM, Kornas K, Fisher S, Hong A, Manuel D, Rosella LC. Development and validation of a population-based risk algorithm for premature mortality in Canada: The Premature Mortality Population Risk Tool (PreMPoRT). BMJ Public Health 2024;2:e000377. doi.org/10.1136/bmjph-2023-000377.
Avoidable Hospitalization Population Risk Tool (AvHPoRT)
We are currently developing a 5-year incidence of first avoidable hospitalization for seven ambulatory care sensitive conditions (angina, asthma, congestive heart failure, chronic obstructive pulmonary disease, diabetes, epilepsy, and hypertension.
Abstract
Introduction
Avoidable hospitalizations are considered preventable given effective and timely primary care management and are an important indicator of health system performance. The ability to predict avoidable hospitalizations at the population level represents a significant advantage for health system decision-makers that could facilitate proactive intervention for ambulatory care-sensitive conditions (ACSCs). The aim of this study is to develop and validate the Avoidable Hospitalization Population Risk Tool (AvHPoRT) that will predict the 5-year risk of first avoidable hospitalization for seven ACSCs using self-reported, routinely collected population health survey data.
Methods and analysis
The derivation cohort will consist of respondents to the first 3 cycles (2000/01, 2003/04, 2005/06) of the Canadian Community Health Survey (CCHS) who are 18–74 years of age at survey administration and a hold-out data set will be used for external validation. Outcome information on avoidable hospitalizations for 5 years following the CCHS interview will be assessed through data linkage to the Discharge Abstract Database (1999/2000–2017/2018) for an estimated sample size of 394,600. Candidate predictor variables will include demographic characteristics, socioeconomic status, self-perceived health measures, health behaviors, chronic conditions, and area-based measures. Sex-specific algorithms will be developed using Weibull accelerated failure time survival models. The model will be validated both using split set cross-validation and external temporal validation split using cycles 2000–2006 compared to 2007–2012. We will assess measures of overall predictive performance (Nagelkerke R2), calibration (calibration plots), and discrimination (Harrell’s concordance statistic). Development of the model will be informed by the Transparent Reporting of a multivariable prediction model for Individual Prognosis or Diagnosis (TRIPOD) statement.
Link to study protocol:
Rosella LC, Hurst M, O’Neill M, Pagalan L, Diemert LM, Kornas K, Hong A, Fisher S, Manuel DG. A study protocol for a predictive model to assess population-based avoidable hospitalization risk: Avoidable Hospitalization Population Risk Prediction Tool (AvHPoRT). Diagn Progn Res. 2024 Feb 6;8(1):2. doi: 10.1186/s41512-024-00165-5. PMID: 38317268; PMCID: PMC10845544. https://doi.org/10.1186/s41512-024-00165-5.
Population Risk Tools at work
Population Risk tools play a crucial role in promoting positive health outcomes, addressing health inequities, and advocating for community-centric policies. By leveraging these tools, public health professionals can make informed decisions and improve the well-being of specific populations.
Our Population Risk tools support several major areas for population health planning:
1. Population risk assessment – Understand the distribution of risk in the population; identify priority groups and population targets for intervention.
Read more about the research here: ● Rosella LC, Kornas K, Green ME, Shah BR, Walker JD, Frymire E, & Jones C. (2020). Characterizing risk of type 2 diabetes in First Nations people living in First Nations communities in Ontario: A population-based analysis using cross-sectional survey data. CMAJ Open, 8(1), E178–E183. https://doi.org/10.9778/cmajo.20190210. |
2. Prevention and Intervention Planning – Test intervention scenarios to estimate the population health benefits of intervention/policy approaches. Population health benefits are estimated by considering the target population size, average baseline risk, relative benefit of the intervention or policy, and intervention coverage.
Read more about it here: ● Chiodo S, Pagalan L, Hurst M, O’Neill M, Stylianou H, Diemert LM, Chen H, Brook JR, Hong A, & Rosella LC. (2024). Measuring the Health Co-Benefits of Air Pollution Interventions on Premature Deaths in Canadian Cities. Journal of City Climate Policy and Economy 2024 2:3, 428-464. https://doi.org/10.3138/jccpe-2023-0019 ● Chen K, Kornas K, & Rosella LC. (2024). Modeling chronic disease risk across equity factors using a population-based prediction model: The Chronic Disease Population Risk Tool (CDPoRT). J Epidemiol Community Health. https://doi.org/10.1136/jech-2023-221080. ● Pianarosa E, O’Neill M, Kornas K, Diemert LM, Tait C, & Rosella LC. (2023). Modelling population-level and targeted interventions of weight loss on chronic disease prevention in the Canadian population. Preventive Medicine, 175, 107673. https://doi.org/10.1016/j.ypmed.2023.107673. ● Kornas K, Rosella LC, Fazli GS, & Booth GL. (2021). Forecasting Diabetes Cases Prevented and Cost Savings Associated with Population Increases of Walking in the Greater Toronto and Hamilton Area, Canada. International Journal of Environmental Research and Public Health, 18(15), 8127. https://doi.org/10.3390/ijerph18158127. ● O’Neill M, Kornas K, & Rosella LC and Wodchis PW. (2021). Estimating Population Benefits of Prevention Approaches Using a Risk Tool: High Resource Users in Ontario, Canada. Healthcare Policy, 16(3). https://doi.org/doi:10.12927/hcpol.2021.26433. |
3. Health resource planning – Estimate future costs and potential savings
Read more here: ● Bilandzic A, & Rosella LC. (2017). The cost of diabetes in Canada over 10 years: Applying attributable health care costs to a diabetes incidence prediction model. Health Promotion and Chronic Disease Prevention in Canada: Research, Policy and Practice, 37(2), 49. https://doi.org/10.24095/hpcdp.37.2.03. |
4. Program scalability – Estimate the population benefit of scaling up new or existing programs
Implementation
Our team developed the Population Health Planning Knowledge to Action (PHPKtoA) Model to support the implementation of PoRTs and other decision-analytic tools in practice. The PHPKtoA model offers a guide for researchers and health system leaders to collaboratively develop relevant analytic tools (Tool Creation Path) and a process to facilitate application of the tools in diverse health settings (Action Cycle Process). Following the PHPKtoA model, we have developed our PoRT tools collaboratively with health system partners and supported the implementation of our PoRTs in several public health units and other health settings in Canada.
To further support deployment and use of PoRTs, we are collaborating with Dr. Birsen Donmez from the Department of Mechanical & Industrial Engineering to apply human factor engineering methods by engaging with end-users to develop an effective user-friendly interface for CDPoRT.
Read more here: ● Vasquez H, Pianarosa E, Sirbu R, Diemert LM, Cunningham H, Harish V, Donmez B, Rosella LC. (2024). Human factors methods in the design of digital decision support systems for population health: a scoping review. BMC Public Health 24, 2458. https://doi.org/10.1186/s12889-024-19968-8. ● Tessema T, Vasquez H, Rosella LC, Donmez B (2024). Public Health Decision-Making Using Uncertainty Displays. Proceedings of the Human Factors and Ergonomics Society Annual Meeting. doi.org/10.1177/10711813241280938. |